What Type of ‘Omics Data Should You Generate?
- 12th November 2020
- Posted by: Claudine Gabriele
- Categories: Articles, Bioinformatics, Biomarkers, Epigenetics, Gene Expression Analysis, Mass Spectrometry, Proteomics, Single Cell Analysis
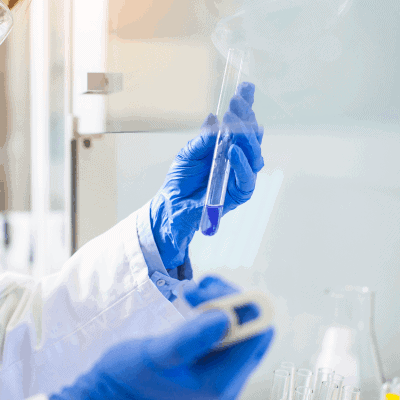
Your research usually starts with a question you want answered or a hypothesis to be tested. The type of ‘omics data you generate, however, will depend on the exact question you are asking. We look into the more common types of ‘omics data generated, and some of the reasons you might prefer them to other data.
Gene expression data
Gene expression gives us a snapshot of a sample’s transcriptome and provides insight into the composition and activity of cells (for example) in a sample. Most analyses of gene expression data are designed to quantify changes in expression between groups of samples that differ with respect to treatment, experimental condition or disease status
Gene expression can be generated in a number of ways such as through RNAseq, microarray, and nanostring data platforms.
RNAseq data generation
RNAseq data can give the ability to look in detail at differential expression analysis, such as changes in gene expression over time or following different treatments.
For example, if you are looking to:
- Analyse expression profiles of drug-sensitive or drug-resistant cell lines
- Assess the effect of a gene knockout on gene expression in cell lines, e.g. of a specific cancer
- Associate drug response with changes in gene expression profiles, e.g. to identify potential mechanisms of response to a certain treatment
- Analyse gene expression of diseased vs. normal samples to gain further insight into the potential pathological mechanisms
- Investigate the effect of drug treatment on the gene expression profile of a cancer cell line over a period of time
- Identify gene signatures that are associated with response to a certain treatment
RNAseq data can be generated to find answers in all the studies above.
Data such as bulk RNAseq, microarrays (commercial and customised), and NanoString gene expression data can all be analysed with bioinformatic techniques, with data being generated in various formats.
Genotyping data
Genotyping allows for the determination of differences in an individual’s genotype, by examining their DNA sequence and comparing it to a reference sequence. It also allows for biological populations, including microorganisms, to be defined.
DNAseq data generation
Why use DNAseq data? For genotyping analysis, DNAseq data can be used analysed to interrogate genetic variation and its association with clinical outcomes or phenotype. DNAseq data can be used to conduct genome wide association studies (GWAS), detect mutations in cells both germline and somatic, as well as analyse copy number variation and SNP mutations.
DNAseq data can be used for studies such as:
- Identifying genomic loci associated with disease status
- Identifying SNPs associated with poorer drug response and survival
- Biomarker assessment (e.g. tumour mutation burden) and its association with response to therapeutics and survival
- Associating copy number variants with cancer cell line gene dependency
- Investigating associations between pharmacokinetic data and genetic variation
Proteomics data generation
Proteomics is another area for specific data generation. Most analyses of proteomics data are designed to quantify the changes in protein abundance, modification, location or binding specificity between groups of samples that differ with respect to tissue of origin, treatment, experimental condition or outcome.
Proteomics analysis can be applied to many different applications, for example:
- Profiling of proteomes between normal and diseased tissues
- Identification and quantification of protein biomarkers and post-translational modifications associated with drug response or survival
- Identification of direct targets and indirect effects of drugs and other active molecules
- Investigation of drug- or gene knockout-induced changes to the proteome of cancer cells or tissues from various model organisms
- Identification of the protein response to drug treatment in blood samples taken from oncology patients
- Correlation of protein levels with patient response to treatment, to identify predictive biomarkers
Proteomics data generated from mass spectrometry (labelled or label–free) can be analysed as well as protein immunoassays.
Metabolomics data generation
The metabolome contains all metabolites in a cell, tissue, or organism which are the end product of cellular processes. Metabolomics analysis also allows for research into interactions taking place in the biochemical networks of a sample.
Metabolomics analysis can be used to generate insights into:
- Drug discovery, development and mode-of-action
- Mechanisms of diseases such as atherosclerosis, cancer and diabetes
- Evaluation of new therapies
Epigenetics data
Epigenetic data can be used to investigate disease mechanisms and develop targets aiming at the epigenome. Methods such as methyl-seq, ChIP-seq, ATAC-seq, and CLIP-seq as well as specific arrays can all be used to generate data for analysis.
Epigenetic analyses give the ability to:
- Gain insight into protein–DNA interactions
- Investigate methylation patterns
- Characterise the response of cells or tissues to epigenetic modifying agents
- Understand the mechanisms that regulate gene expression in response to environmental stresses or during developmental processes
Single-cell data generation
Single-cell data is becoming a more common method for data generation. All types of ‘omics data can be analysed during single-cell analysis using both high- and low-throughput screening methods, meaning it is a very versatile method of data generation.
For example, if you are looking to:
- Analyse healthy vs diseased cells of a specific cancer from a large cohort of patients
- Evaluate gene expression changes in response to treatment in multiple types of cells from tissue samples of the same type
- Associate gene expression and functional pathways with immune cell subtypes in specific cell types
Using single-cell data generation can find answers in all the studies areas above – and more.
Use of public data
It might not always be the case that you want to generate your own data. There is a wealth of data stored in public databases that can be landscaped and mined for further research. Using publicly accessible data – or even datasets in-house that can be further analysed – can reduce the cost for research. There is no data generation cost for you, as the data has already been generated.
Public data sources contain many different types of data (including gene expression, proteomics, and metabolomics). They can also help with repositioning your therapeutics, reducing both the time and cost needed when starting in a new area of speciality.
For oncology-related data, there are many datasets and resources, such as:
- The Cancer Genome Atlas (TCGA)
- Cancer Dependency Map (DepMap)
- Cancer Cell Line Encyclopaedia (CCLE)
- cBioPortal for Cancer Genomics
Other commonly used repositories for publicly accessible data include:
- Gene Expression Omnibus (GEO)
- European Nucleotide Archive (ENA)
- Expression Atlas
- Database of Immune Cell EQTLs, Expression, Epigenomics (DICE)
Leave a Reply
You must be logged in to post a comment.