Are You Making The Most Of Your Drug Development Data?
- 18th November 2021
- Posted by: Breige McBride
- Category: Uncategorised
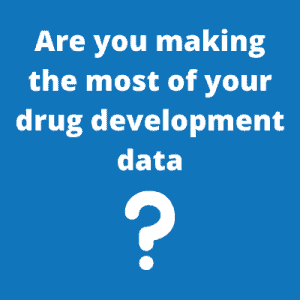
We live in a very data-thirsty era. We are consuming, and indeed creating, data every minute of every day. Some data is for personal use as we strive to achieve targets we set ourselves (e.g. steps, macros, share price). However, for those working in drug development, the generation and accumulation of development data creates issues as well as opportunities.
Drug Development Data and Accessibility
The target most developers aim for is to get a safe and effective product into patients and on the market as quickly as possible – or certainly to obtain proof of concept in order to partner the asset. For a single drug that enters a Phase II trial, the amount of data that will have been generated from lead ID/validation through to that point is immense. This data will also be multi-dimensional in nature (to say the least!) and generated over anything from 5-10 years.
For most companies, the data generation approach is likely a hybrid between in-house facilities with augmentation by contract research services. While they can upload the in-house data centrally via a LIMS system, data and reports from multiple CROs may have arrived via email, hard drives, or electronically by secure download links. It is possible that data for any particular assay or from a particular vendor may have been received by 1 person who may or may not be with the organisation for the duration of the drug development process. It is easy to see how things can get lost or forgotten about.
As well as the “where is…” issue, most of us will have experience of the “what is…” problem. Excel files uploaded to a common drive with a non-descript title. For example, a title containing a matrix of numbers, perhaps a concentration level, and a cell line name or two. Or perhaps a file with no obvious extension that only opens with a software someone once had on a laptop. Whilst the lack of consistency and clarity can be an irritation in everyday working, it highlights the much greater problem of organisations not looking after perhaps the second biggest asset that they have.
What is your data telling you?
In addition to data house-keeping issues, sometimes organisations do not make the most of the data as it is being generated. In recent times the ability to generate data has far outstripped our capacity to analyse and digest the results. Added to this lack of capacity is a lack of data standardisation within organisations, making the situation worse.
It is common that once researchers observe the primary data output of an experiment (for example efficacy data from a PDX model) they will forget about the secondary data that is available (for example gene expression or mutational profiling data from the PDX). This is often the case when the primary endpoint fails, creating the perception of “throwing good money after bad”. Similarly, you may also observe this at the clinical stage of drug development when there is a decision to pull assets from development after they fail to reach their primary endpoint. Whilst understandably this can have significant financial consequences for organisations, where possible it can be very beneficial to complete the analysis plan including all data types to try and salvage something from the trial to take any learnings forward – especially as no one goes into a trial thinking it will fail.
What to consider when primary endpoints fail
When the primary endpoint fails, we can still gain valuable insights by considering questions like the following:
- Was the endpoint selection incorrect?
Overall response vs progression-free survival etc.
- Was there a subpopulation of patients that did respond?
Was there an opportunity to identify these patients pre-treatment?
- Were the exposure levels high enough to elicit a response without causing safety issues?
The PK profile of an agent may be quite different in patients vs healthy controls
If limiting, was there an opportunity to identify on-target-off tissue or off-target effects earlier?
Discovering answers to the above questions is especially important if you have follow-on assets with a similar target/structure or are working in the same indication. Alternatively, having more information about asset performance in a diseased population may open up new avenues for development through drug-repositioning. This ensures that the investment in clinical development is not a complete write-off.
How to make the most of your drug development data?
Hindsight is a wonderful thing during the evolution of every organisation and no one wants to be process- or bureaucracy-heavy, particularly in the early days. However, ensuring your data is RETAINED, ACCESSIBLE, DESCRIBED, INTEGRATED, CATALOGUED, ANALYSED and LEARNED from is not too radical a concept.
From day 1 have a data retention and curation process in place. Ensure centralised storage of raw and processed data from your labs or external vendors as well as the final reports documenting the rationale, methodology and top-level outcomes of the work. Don’t allow anyone to save data on laptops or in email accounts. This way, you are not at risk of losing it when employees move on (or when the blue screen appears).
Standardise your data
Where possible have a standardised format across the organisation for data storage for each particular modality – like Next Generation Sequencing for example. This will make the creation of an in-house data lake/reservoir/loch/warehouse much easier down the line. Many companies are now spending a lot of time retrospectively re-analysing data in a consistent manner to generate huge repositories of their own data coupled with public domain data to create data rich resources which they can use at all stages of development. Ensure upfront that the vendors you are working with can accommodate your specific requests for formatting. This is important as it can be expensive and time-consuming to “retro-fit” later.
Make your data searchable
For all pre-clinical drug development experiments, ensure that a descriptive file that can be associated with the data is available. This file should outline the aims, the model used, concentrations/doses, and controls. It is also useful to ensure it contains a list of all the data generated.
In the absence of a formal data repository, ensure that there is a searchable list or catalogue of all the experiments and data you have generated available. This helps avoid duplication of effort (at Fios we often receive requests from different people in the same organisation to analyse the same data set). A searchable list also facilitates data re-mining; allowing other PIs at your organisation to make use of the data to ask different questions.
Whilst the documentation for clinical analysis is usually more formal and robust than pre-clinical, ensure you give any exploratory clinical data sets the same due care and attention as the formal read-outs of the trials.
Reveal all of the insights from your drug development data
Ensure, where possible, that you fully analyse the data you generate in experiments and trials. Do this even if the primary endpoint of the experiment or trial is not successful. Post-mortems of clinical trials can often identify the reasons for the failure and help obtain useful information to carry forward to future programmes.
Last, but certainly not least – learn from the data your organisation has created. Don’t be afraid to revisit and re-analyse old data. The approaches and tools that turn data into information have evolved just as much as the data generation technologies themselves. Researchers are increasingly turning to public domain data to add more information to their programmes. However, they often forget that the most relevant data will have been generated in-house.
While the above measures are not exhaustive; it is important that how you deal with the drug development data you will generate is not an afterthought. All of the above will take investment both in time and money; However, when compared to the investment made in generating the data, perhaps you simply cannot afford not to.
See also:
Video: How to get the most out of your data
Leave a Reply
You must be logged in to post a comment.