Advancing AAV Gene Therapy Research With scRNA-seq
- 19th February 2024
- Posted by: Breige McBride
- Categories: Bioinformatics, Cell and Gene Therapy, Single Cell Analysis
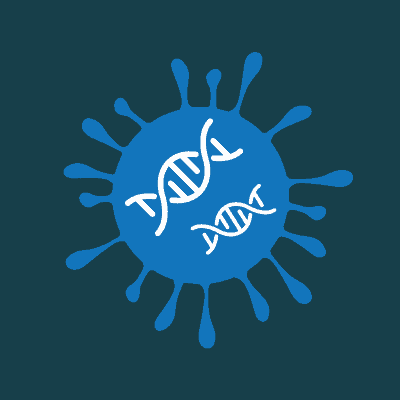
AAV gene therapy refers to gene therapies which use Adeno-Associated Viruses (AAVs) as vectors to deliver the therapeutic genes into target cells. Since AAVs trigger a minimal immune response and can infect a wide range of cell types, they are a popular choice for gene therapy vectors. Here we will look at how analysis of single-cell RNA sequencing (scRNA-seq) data supports AAV gene therapy research.
Applications of scRNA-seq Analysis in AAV Gene Therapy Research
Identifying Target Cells
Researchers can use scRNA-seq technology to assist with identifying the specific cell types involved in disease pathology, or other cells that are potential targets for the gene therapy. Analysis of gene expression profiles at the single-cell level can pinpoint the most suitable cell populations for AAV vectors to deliver therapeutic genes to.
Characterising Disease Mechanisms
scRNA-seq analysis allows the identification of the gene expression patterns and regulatory networks in diseased cells. This helps researchers to gain insight into the molecular mechanisms which contribute to disease development and progression at the cellular level. Subsequently, this helps them to design AAV gene therapies that effectively modify the molecular mechanisms of the disease and alleviate disease symptoms.
Optimising Vector Design
In order to ensure efficient and specific gene delivery, the AAV vectors need to be carefully selected and optimised for gene therapy. This is something that scRNA-seq data analysis also assists with. Analysis of scRNA-seq data can reveal insights into the expression levels of various surface receptors and intracellular signalling molecules in target cells. In turn, these insights can guide the design of AAV vectors with enhanced tropism and transduction efficiency.
Assessing Treatment Efficacy of AAV Gene Therapies
Once an AAV gene therapy has been administered, researchers can evaluate the impact of treatment at the single-cell level via scRNA-seq analysis. Since scRNA-seq analysis enables comparison of the gene expression profiles of treated and untreated cells, it allows researchers to assess the therapeutic effects of the treatment. For example, they can assess whether normal gene expression patterns are restored. They can also check if there has been modulation of disease-associated pathways.
Predicting Treatment Response and Resistance
scRNA-seq data analysis can help predict individual patient responses to AAV gene therapy and identify potential mechanisms of treatment resistance. Insights gained by analysing the gene expression profiles of patient-derived cells guide patient stratification. Essentially, scRNA-seq data analysis enables researchers to stratify patients in relation to their cellular gene expression profiles and tailor treatment strategies accordingly.
Advancing Your AAV Gene Therapy Research With scRNA-seq Data Analysis
At Fios Genomics, we consider ourselves experts in scRNA-seq data analysis. In fact, at the Festival of Genomics in 2022 we presented a workflow for analysing single-cell RNA sequencing data. We have also written a whitepaper about single-cell analysis applications and resolutions for research.
So, if you need support with scRNA-seq data analyses to advance AAV gene therapy research, we can certainly help you. Contact us now to discover exactly how we can support your particular research.
Prefer to see what you can expect from a Fios Genomics scRNA-seq data analysis report before contacting us? No problem, you can request our sample scRNA-seq data analysis report via the form below.
Request a sample of our scRNA-seq data analysis reports
This report describes the analysis of a single-cell RNA sequencing (scRNA-seq) dataset containing peripheral blood mononuclear cell (PBMC) gene expression profiles sampled from a healthy donor. This dataset was obtained from the publicly available 10X Genomics download portal.
In brief, the main objectives of this analysis were to:
- Identify cell clusters in the data and annotate these by immune cell type using established biomarkers.
- Identify differentially expressed genes (DEGs) between cell populations.
- Identify functional pathways that are significantly enriched in DEGs between clusters.
This report showcases our data analysis report structure and capabilities in Single-Cell RNAseq analysis.
Fill out the form in order to access the report.
Author: Breige McBride, Content and Social Media Manager, Fios Genomics
Reviewed by Fios Genomics Bioinformatics Experts to ensure accuracy
See also:
Bioinformatics Analyses for Cell and Gene Therapy Research