Benefits of RNA sequencing
- 25th July 2022
- Posted by: Claudine Gabriele
- Categories: Bioinformatics, Gene Expression Analysis
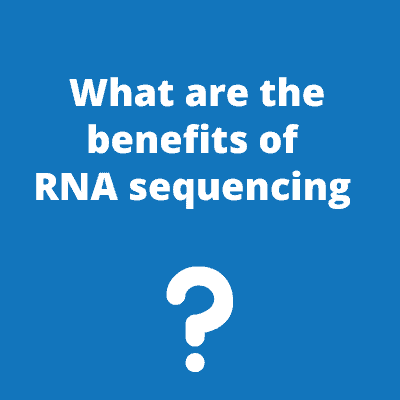
What is RNA Sequencing Used For?
RNA sequencing (in short, RNA-Seq) is widely used to analyse the transcriptome of a cell and its changes. If the goal is to add to the analysis of gene expression, researchers can perform RNA-Seq on its own for transcriptome profiling. For other purposes, such as gaining insight into RNA-protein binding or the structure of RNA, researchers can use RNA-Seq alongside other assays.
Researchers can use RNA-Seq for a wide range of goals, including:
- Profiling and quantifying gene expression
- Discovery of novel transcripts
- Identifying alternatively spliced genes
- Detecting allele-specific expression
There is a variety of methods for performing an RNA-Seq experiment. In fact, the techniques are evolving so rapidly that it can be difficult to decide which one to use. A very basic choice is between 1) random-primed cDNA synthesis from double-stranded cDNA, which is the most used, or 2) RNA-ligation methods (reviewed and compared in Levin 2010).
Benefits and Challenges of RNA-Seq Data
If we compare it to microarray, RNA-Seq provides higher coverage and resolution of the ever-changing nature of the transcriptome. There are other benefits:
- RNA-Seq allows far higher level of reproducibility compared to microarray. This means that once they obtain more information about the transcriptome, researchers can re-analyse RNA-seq datasets
- There is no use of primers or probes in RNA-Seq, and therefore less biases appear in the data. However, this is not to say that RNA-Seq has no biases at all
- RNA-Seq improves the chance of detecting rare transcripts as it provides aligned read counts and has a very wide dynamic range for detection
Alongside these benefits, RNA-Seq also presents challenges. Some of these challenges are connected to the wealth of RNA-Seq data:
- Complexity in extracting the most meaning from RNA-Seq datasets because of the volume of data
- Risk of misinterpretation of these data, if the correct expertise and skills are not available
- Need to integrate the RNA-Seq data validation with other methods (such as qPCR)
- Requirement for an available reference genome.
At the moment, the analysis of these data presents some challenges as well, despite the large availability of basic analysis tools.
Analysis of RNA-Seq Data: Common Steps
The standard workflow for RNA-Seq data starts with generating FASTQ files: these contain reads sequenced from an NGS platform. Researchers then align the reads to an annotated reference genome and consequently quantify the expression of genes.
At Fios Genomics, our team includes the following steps in a typical RNA-Seq data analysis workflow:
- Quality control evaluation of raw gene expression data
- Alignment and/or quantification using standard bioinformatics tools (e.g. STAR, TopHat, salmon etc) as required
- Normalisation across samples using trimmed mean of M-values normalisation (TMM)
- Analysis of sample metadata to identify, for example, associations between biological and technical variables
- Exploratory data analysis and evaluation using unsupervised clustering and dimension reduction techniques to assess overall sample quality and identify possible outliers or batch effects
- Differential expression analysis with a range of tools (e.g. voom/limma, DESeq2, EdgeR)
- Functional enrichment analysis using curated resources such as the Reactome pathway database and the Gene Ontology (GO) resource.
Our team can also provide similar pipelines covering all array platforms. They can tailor these pipelines and apply the most appropriate techniques in any situation, e.g. Robust multi-array average (RMA) normalisation for Affymetrix data.
Furthermore, if required, we can include a range of further bolt-on analyses, for example, gene set enrichment analysis (GSEA) to assess functional enrichment. You can find more about our offering here.
When you arrive at the analysis stage of your research, you may need to outsource the analysis to a bioinformatics provider. At Fios Genomics, we have a great deal of experience in this area. Our skilled bioinformaticians possess the biological knowledge necessary to understand and interpret your transcriptomics data to provide biologically relevant insights to further your research.
To discuss your next transcriptomics analysis project, contact us now!
You may also be interested in:
Our Bioinformatics Services
Our Data Analysis Reports from Fios Genomics
Fios Genomics on YouTube
Download RNASeq Data Analysis Report
Fill in the form below to access the data analysis report our team created. Here, bioinformatics analysis of publicly available RNA sequencing (RNASeq) datasets helped to identify genes and pathways associated with psoriasis and the response of psoriatic skin to modulation of the AhR.
Leave a Reply
You must be logged in to post a comment.