Data Landscaping: How To Guide
- 6th August 2020
- Posted by: Claudine Gabriele
- Category: Articles
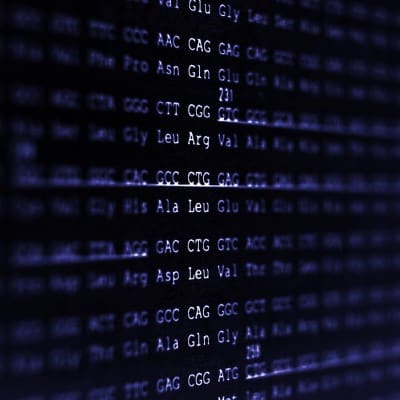
Have you undertaken data landscaping projects before, or are you just starting and want to find out more about how to go about conducting them? We asked some of our resident Bioinformaticians some key questions about data landscaping.
How do you start looking for the right type of datasets?
To start with, it can be as simple as an automated query of the relevant databases using carefully selected keywords. Relevant databases can be well-known ones such as ArrayExpress, or found through searching online.
When landscaping for a client, use their exact criteria, such as any particular genes, diseases, data types, or specific terms that should appear in an associated publication. By formulating a list of search terms, you can capture the nature of the question as fully as possible. Carefully curating a list of search terms should allow for the return of a comprehensive collection of datasets while retaining as much specificity as possible. This ensures that the need for downstream filtering is minimal.
There are many databases out there for ‘omics data. Many specialise in particular data types, however there are some that collate more general collections. The databases you choose to query will depend on the type of data that is of interest to you.
A search could return thousands of datasets. Therefore, it is important to collect as much further information on each dataset as possible to allow for further filtering at a later stage. After this, you can search the datasets for the right data type and species.
What do you look for in a dataset?
The first thing to look for is if a dataset matches all the criteria laid out. If there are many datasets, prioritise them according to the search criteria. For example, if the client is looking for datasets where a particular gene is expressed, give precedence to results where the gene is most highly expressed.
When choosing publicly available data, it is important to look at whether the sample types and treatments they are subjected to will allow the question of interest to be addressed. Ideally, well-annotated datasets containing information such as disease, tissue type, number of samples, will be used.
As a more general guide, the more samples the better. Using datasets from compatible platforms enables the validation of findings in a different data set and also enables the merging together of datasets. Some resources contain several datasets that have already been aggregated, pre-processed and normalised together. You can also filter by different stages of data processing. Raw data will allow reprocessing of several datasets together, however, processed data can save on processing time and cost.
What are the next steps in data landscaping after finding suitable datasets?
After finding suitable datasets, checking the availability of the data at different processing stages is key. This will usually dictate what datasets you can use. After assessing data availability, it is time to share the list of datasets with the client. The datasets can then start to be prioritised for further study as required.
How do you present data landscaping findings?
For ease, we suggest presenting curated results in a table format with the pros and cons of each dataset as well as highlighting any interesting results and recommending which datasets to prioritise. Where a search returns many datasets, it is best to organise these into subgroups to digest the information more easily.
For Fios specifically, our tables are searchable with all the associated metadata included such as title, description, database, species, sample number, and data type.
What challenges do you normally face?
There is a plethora of publicly available data that represents a vast resource. As it grows it becomes increasingly difficult to find what you are looking for. There is no single centralised database and so you have to search from multiple sources. In addition to searching in many places, not every researcher makes their data available. You may find a perfect match from a publication, but there is no publicly available dataset in tandem.
The volume of results is also challenging from an assessment point of view. This is because there is a limit to what it is possible to conduct programmatically before manual curation needs to take over, when the number of datasets found is more reasonable. If the target being searched for is a rare disease, there are likely a reduced number of datasets available, particularly if the search criteria used are strict.
With data available from many different databases, there are challenges with overlapping datasets and amalgamation from different repositories. Databases are also unique in the way they collect their information. The format in which data is retrieved is different for each database. This means that the approach for each needs to be slightly different. Also, even though data formats are usually consistent within databases, this is not always the case. This can sometimes lead to key information being missing or not being in the place you would expect.
There can also be problems with downloading from databases, as well as missing metadata from uploaded data. Sometimes it may not be clear exactly what the dataset contains, and how it matches up with any associated publication.
Why use data landscaping?
Why reinvent the wheel? Alongside other benefits highlighted in our previous blog, it can be cheaper and faster to search for data to answer your scientific question than it is to generate your own data. The sheer scale of publicly available data is growing year-by-year and many public datasets will not have been used to their full potential by their creators.
Analysis of these datasets can help towards gathering evidence at the beginning of a study for the formation of scientific questions, or for corroborating or further investigating observations made throughout the study. You may want to understand the landscape of previous research for a specific target (such as a gene or disease) before setting up your own experiment and research plans. Finally, you could conduct in-silico analysis on combined datasets in the public domain alongside your already-generated data.
We approach data landscaping in a systemic way, to pinpoint datasets that will be most useful to further your research.
Interested in learning more about our data landscaping services? Find out more on our dedicated page and get in contact with our team today.
Read more
Leave a Reply
You must be logged in to post a comment.