How Important is Experimental Design?
- 20th November 2018
- Posted by: Claudine Gabriele
- Category: Articles
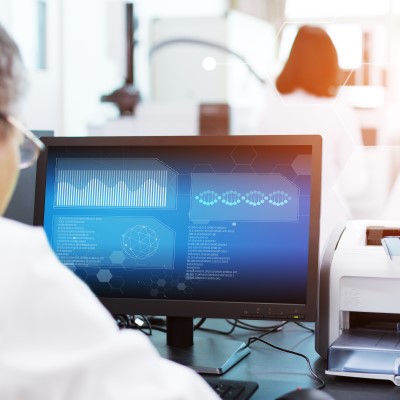
How Important Is Experimental Design?
Experimental design is crucial, especially when trying to avoid bias. You need to set up your study in a way that it will result in not only interpretable outcomes but also useful ones. The latter can then be taken out for data analysis.
Generally, the analysis is the hardest step. However, ensuring your study is well-designed from the outset can reduce issues further down the pipeline, especially at this last stage.
Common Challenges
There are two common issues that occur when experimental design is not given enough consideration:
- Not having enough replicates
The first thing to remember is that without replicates, there is a chance that results will be invalid. This could be due to error or just by chance. Results from studies need to be reproducible in order to ensure validity. Also, with statistical analysis, multiple replicates allow for trends or patterns to be properly identified. - Single batch creation
When you have an experiment with two subtypes, such as control and treatment variables, running all of one type in one go can add another variable to the analysis. While it is not the easiest way to run an experiment, changing between different subtypes will ensure that batch effects are not a factor.
How To Ensure A Good Experimental Design
Having a good design from the start can help with results further down the analysis pipeline. Good experimental design generally has:
Sufficient replication
Ensuring that you have enough replication in your experiment will markedly reduce the variability in your results. This will increase their significance and also the confidence level that a researcher is able to draw conclusions on. Therefore the sample size needs to be large enough to detect changes. As a matter of fact, you can work out the optimum number of samples through formulas for each experiment.
Randomised sampling
Samples can be randomised at two points of an experiment, firstly when subjects are assigned to treatments and secondly when samples are run. When assigning treatments, a completely randomised design supposes that external factors affect the results equally. If, however, full randomisation cannot be achieved or would not be suitable for your experiment, using block randomisation is another method to avoid unwanted associations. Block randomisation groups subjects by other factors before they are assigned to a specific treatment, such as by age or gender if that may factor into how a treatment performs.
When samples are run, ensuring this is done in random order (and not doing all of one type of sample in one go) helps avoid associations between technical and biological factors. This ensures that factors such as operator fatigue or daily variation do not cause batch effects on samples.
Consistent measurement methods
Results should be both accurate and precise. It is important to realize that these two terms are subtly different. Accuracy is being close to the ‘true’ results, while precision is having good repeatability or reproducibility. For example, an experiment can give good precision, with all results close to each other, yet be inaccurate and far from the ‘true’ results. A lack of accuracy or precision can be due to differences in operators or equipment calibration. However, these factors can be reduced with good quality control during the experiment run.
‘Blind’ experiments
Most common in clinical trials, ‘blind’ experiments are important to ensure that researchers do not know which treatment or trial they are observing, in order to reduce unconscious bias. They are also important when working with live subjects such as humans, so influences like the placebo effect are reduced. While this can make experimental logistics more challenging, it ensures results are not influenced, making them more reliable.
Above all, placing your research question at the centre of your experimental design is key. By ensuring that your experiment is planned in the first place to be able to answer the specific questions posed, the right output can be created to answer your questions.
Fios can come on board at any stage of a project to assist where needed – whether to give advice on how to set up your studies or to guide decisions later in the process. To learn more about how we can help with your experimental design in particular, watch our video below. Of course, you can also contact us. If you have any questions then just let us know and we will be happy to answer them.
Access our Data Analysis Reports
Fill in the form below to access a demo of our bioinformatics data analysis reports. The demo showcases bioinformatics analysis of publicly available RNA sequencing (RNASeq) datasets. These helped to identify genes and pathways associated with psoriasis as well as the response of psoriatic skin to modulation of the AhR.
Leave a Reply
You must be logged in to post a comment.