Fios at the Festival of Genomics and Biodata 2022
- 7th February 2022
- Posted by: Breige McBride
- Categories: Events, Sequencing, Single Cell Analysis
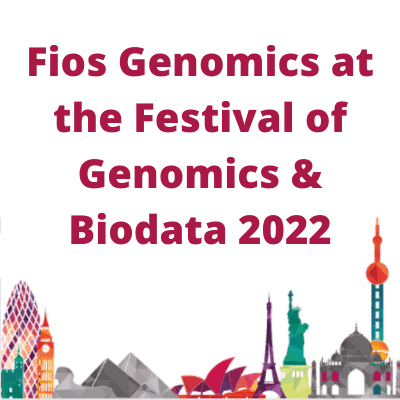
The Festival of Genomics and Biodata is the UK’s largest genomics event; drawing attendees and speakers from all over the globe. The festival covers a multitude of topics, ensuring that there is something to attract everyone who works with genomics data, no matter their research area.
This year’s festival was a 4-day virtual event which took place from the 25th-28th of January. With over 200 speakers this year, there was a lot going on. Popular topics included nationwide screening programmes, cancer genomics and, of course, bioinformatics. As experts in bioinformatics analyses for genomics data; we are always happy to share some of our expertise at the festival by hosting a talk of our own.
Talking About Genomics Data: An Analysis workflow for single-cell RNA-sequencing data
On Day 3 of the festival, Fios bioinformatician Katerina Boufea discussed an analysis workflow for single-cell RNA-sequencing data. You can view this talk below. Topics Katerina discusses include quality control, clustering and differential gene expression analysis.
Following her talk, Katerina answered the following viewer questions:
Q: Do you have any recommendations on downstream cell type labelling approaches? Also, how does performance of these relate to technical biological variations you mentioned?
A: The most commonly used approach is to manually explore the expression of cell type specific markers. However, this can be time-consuming and requires expert knowledge on the cell types studied. There have been quite a few methods for automated cell type labelling. For reference, I am providing links to two interesting review papers. This paper shows a thorough comparison of the performance of these methods across multiple datasets while this paper is a more recent brief overview of the latest methods.
The majority of these methods follow a transfer learning approach where they expect a reference dataset with annotated cell types exists that we can use to learn discriminatory features for these cell types and use these to annotate the new cells. These can work well in some cases, although their performance depends heavily on the dataset.
A practical challenge with this assumption is that it is difficult to have a publicly available good quality reference dataset for all tissues we may want to analyse. Additionally, my concern is that when a cell type is present in the new dataset but it is absent from the reference, it is possible that these cells are assigned to the most transcriptionally close cell type present in the reference dataset rather than left unassigned. So, the bottleneck is not really the methods but good quality datasets that we can use to define all possible cell subtypes/states in a tissue. Hopefully, the human cell atlas project will provide such data and resolve this issue for human samples.
Q: I have noticed that sometimes samples coming from the same dataset even without batch correction, usually formed clusters based on the cell types. However, if I tried to extract a specific type of cells (for example malignant cells) from these samples and do clustering analysis without batch correction, usually I get clusters based on the samples, not on the characteristics of the cells.
A: Batch effects typically arise from samples that have been processed separately (i.e. come from different datasets). You are right that batch correction is unnecessary for cells from multiple samples that are processed as a single dataset. What you observe for the malignant cells is expected. Malignancies tend to be different between individuals which leads to different transcriptional patterns between individuals. Thus, it is common for malignant cells from different patients to cluster by patient ID as they are indeed in different states.
Q: Which statistical tools would you recommend for differential gene expression analysis of scRNA-seq data? Does sparsity of the data impact statistical modelling at all?
A: Sparsity does affect statistical testing methods used for differential gene expression analysis in bulk RNA-seq data. The issue arises from the uncertainty of the meanings of zero values. Some zero values indicate that a gene is not expressed in the cell; but it may also indicate that a gene was not captured for sequencing (dropout events). For this reason, my recommendation is to use a method that not only models the distribution of gene expression across all cells of each group; but also takes into account the proportion of zero values. One such method is MAST, developed specifically for scRNA-seq data. MAST tests the distribution of the non-zero expression values of a gene between two groups of cells and the difference in the proportion of zero values of the gene across the cells.
What got the Fios office talking
With so many talks during the festival, there were a lot of interesting projects and techniques for genomics data to learn about. Here are a few things that got the Fios office talking:
Lift off for Project Maleth – Malta’s first genomics study in space.
University of Malta Associate Professor, Joseph Borg, presented this talk.
Professor Borg discussed the prevalence of diabetes in Malta, which is the highest in Europe, before detailing how and why his team sent six samples from Diabetic Foot Ulcers (DFUs) to the International Space Station. The project aim is to study how space travel conditions affect the microbiome of DFUs to learn about the pathways or mechanisms of antibiotic resistance. You can learn more about this out of this world project here.
The Future of Genomics: Health and Beyond
Chief Scientific Adviser to the UK Government, Sir Patrick Vallance, presented this talk.
Sir Patrick discussed advances in genomic sequencing in terms of speed, cost, depth and accessibility. He then discussed how advances in our ability to analyse genomics data are changing the entire field. Later in the presentation, he discusses the wider implications for society with the increasing availability of genomics data and our increasing capabilities to interpret it. For example, he mentioned how this could affect things like the insurance industry. Throughout the presentation, he referenced a UK Government report, Genomics Beyond Health. This report discusses the potential future uses of genomics data beyond health as well as various other genomics-related topics.
See also
Single-cell data analysis from Fios Genomics
Predictions for bioinformatics in 2022
Leave a Reply
You must be logged in to post a comment.