Optimising Preclinical Drug Development with Data Mining
- 17th April 2024
- Posted by: Breige McBride
- Category: Data Mining
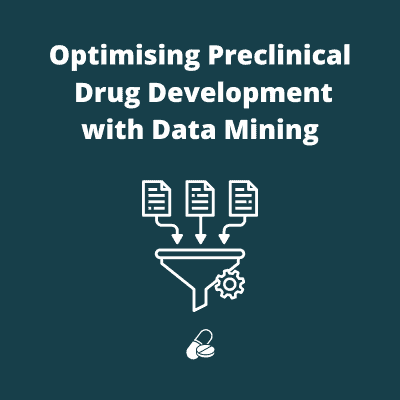
The overall goal of preclinical drug development studies is to gain regulatory approval to begin human clinical trials of a drug. This approval is often in the form of a successful Investigational New Drug (IND) application. Submitting an IND application is the end of the formal preclinical phase of drug development.
However, there is a lot of work to do before that.
This work begins with research and development to identify a potential drug, in the discovery phase. Data mining isn’t always a part of this phase, but it can bring significant benefits.
How Can Data Mining Optimise Discovery?
Target Identification
At Fios Genomics, we believe target identification should start with data mining. This is because we can use data mining methods to search through literature and databases relevant to the disease to be treated, to find insights to facilitate target identification. For example, we have previously mined the Parkinson’s Progression Markers Initiative (PPMI) database for information to help identify drug targets. We use data mining to analyse things like gene expression profiles, biological pathways, protein-protein interactions, and various other types of omics data, specifically to identify drug targets. Of course, we can also ‘mine’ any data previously generated in-house, for similar insights to optimise this stage of preclinical drug development.
Target Validation
The aim of target validation is to confirm if, when the drug target is altered, either by being knocked out or activated, this impacts the disease to be treated either by halting or slowing progression and/or by alleviating symptoms. This is another area of preclinical drug development where data mining is beneficial. We can use data mining to analyse publicly available data for insights into the underlying biological mechanisms involved in disease pathology, to optimise target validation. By understanding such mechanisms, we can confirm if the target is relevant to the disease, and how it can be acted on to alter the disease.
We can also analyse any in-house data to assess if a target behaves as expected. For example, we can analyse CRISPR screens, knockout models, or gene expression data to find out how a target behaves when altered.
Why is Data Mining Beneficial at the Pharmacology Stage of Preclinical Drug Development?
Data mining is beneficial at the pharmacology stage (before preclinical safety and toxicology studies) for a variety of reasons. For example, by mining preclinical data such as in vitro pharmacological assays, we can make predictions about a new drug candidate’s safety and efficacy. A more specific example of data mining being beneficial at the pharmacology stage of preclinical drug development, is mining datasets which contain information about biological pathways and known drug interactions to predict potential off-target effects.
In fact, data mining also helps to identify on-target off-tissue effects. For example, by analysing gene expression profiles across different tissues and cell types, data mining can help identify potential off-tissue effects by predicting where a drug might exert unintended effects.
Also, data mining in the pharmacology stage can help make predictions about the toxicity of drug candidates. This is because we can use data mining approaches to analyse structure-activity relationships, as well as integrate toxicological data (from various sources) into these analyses. As a result, researchers can determine which drug candidates have discouraging safety profiles and prioritise safer candidates. In fact, data mining can even guide the development of targeted therapies. For example, mining gene expression data from healthy and diseased tissues can help identify disease-specific alterations in the expression profile of the target, informing precision-based therapies.
We can also use data mining techniques to create pharmacokinetic models that predict the ADME properties of drug candidates. Such models can help with optimising drug dosing regimens and predicting plasma concentration-time profiles.
What’s more, data mining helps researchers to optimise their experimental design before proceeding to pharmacological studies. Mining experimental data reveals insights which can inform study design and hypothesis testing. In fact, by using data mining to identify the key variables and experimental conditions that impact study outcomes, researchers can improve the efficiency and reproducibility of their preclinical experiments.
Optimise Your Preclinical Drug Development With Data Mining
As you now know, there are many ways to optimise preclinical drug development with data mining. These include ways to optimise target identification, target validation and formulation development, as well as ways to optimise pharmacology research. By optimising each of these steps in preclinical development, data mining can significantly accelerate the drug development process and reduce the risk of late-stage failures.
If you would like to optimise your own preclinical development, in any of the ways above, Fios Genomics can help. As a leading bioinformatics analysis provider, catering to the biotechnology and pharmaceutical industries, we have vast experience in data mining. In fact, we provide a wide range of bioinformatics services to reveal insights from both preclinical and clinical data sets.
To discuss a preclinical or clinical data mining project, or any of our other services, contact us today. We are always happy to advise on the most suitable analyses to advance your research.
More information about our data mining capabilities is also available on our Bio Data Landscaping and Mining solutions page.
Author: Breige McBride, Content and Social Media Manager, Fios Genomics
Reviewed by Fios Genomics Bioinformatics Experts to ensure accuracy
See Also:
Drug Repositioning with Gene Expression Analysis
How to Reduce Animal Testing With Bioinformatics