Tool Selection for Cellular Deconvolution
- 28th March 2025
- Posted by: Breige McBride
- Category: Bioinformatics
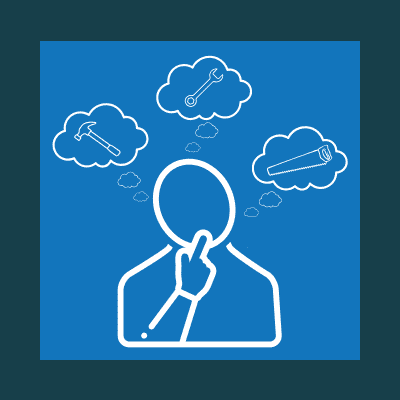
Cellular deconvolution
A common limitation of bulk RNA-sequencing (RNA-seq) studies is that tissue samples can contain significant cellular heterogeneity, hindering our ability to detect cell-type specific gene expression changes.
While single-cell RNA-seq (scRNA-seq) allows analysis of gene expression at the individual cell resolution, it is also possible to estimate cellular composition of a bulk RNA-sequencing sample using cellular deconvolution. This approach allows monitoring of changes in cell population abundances under different conditions, such as disease progression or treatment responses.
For example, the human immunodeficiency virus (HIV) targets and destroys CD4+ T cells, whilst CD8+ T cells expand in response to the virus. Therefore, the CD4+/CD8+ ratio in peripheral blood is a clinical marker for immune dysfunction in HIV (Ron et al., 2024, Front Immunol), and changes in this ratio can be estimated in bulk RNA-seq data sets via cellular deconvolution.
There are many cellular deconvolution tools available (Nguyen et al., 2024, Nucl Acid Res). For example, the following are among the most widely used in the literature:
In this blog, we discuss key factors — such as the reference signature matrix, cell populations of interest, and analysis comparisons of interest — which should be considered when selecting an optimal cellular deconvolution tool.
Reference signature matrix
Cellular deconvolution requires two inputs: a target for deconvolution, typically a matrix of normalized RNA-seq expression counts from a sample panel; and a reference signature matrix representing the cell populations of interest.
The reference signature matrix can be either a matrix of cell-type specific gene expression profiles (GEPs; used to build a linear model by true deconvolution methods such as quanTIseq) or a list of cell-type specific marker genes (used to calculate an abundance score per cell population, such as in xCell or MCP-counter).
Most tools have an embedded reference signature matrix. These typically result from a rigorous process of signature selection from thousands of samples, often from multiple independent projects, followed by validation against existing tools and/or purified samples. These signature matrices are species-specific; for example, human in MCP-counter, quanTIseq and xCell, and mouse in DCQ, mMCP-counter and Seq-ImmuCC. Species should be considered when choosing a cellular deconvolution tool, as reference signatures derived from one species cannot reliably be applied to deconvolute expression in another species.
Alternatively, a custom signature matrix can be used to study specialised or granular cell populations, or species lacking reference matrices. For instance, MuSiC generates a custom GEP matrix by averaging gene expression across cell populations from annotated scRNA-seq data, assigning greater weighting to genes with low cross-sample variation.
Population of interest
Another key factor to consider when selecting a cellular deconvolution tool is the specific cell populations included in its reference signature matrix.
For example, xCell contains a highly granular set of 64 cell populations, based on >6,500 signatures from >1,800 purified samples. In contrast, MCP-counter only contains 12 reference cell populations, retained after a robust signature selection procedure using cell population-specific and stably expressed transcriptomic markers. This validation process produced high confidence signatures, but at the expense of reliable signatures for common cell populations such as CD4+ T cells.
Achieving a balance between granularity, specificity and reliability in the reference signature matrix is challenging. A common issue in cellular deconvolution is crossover between the expression profiles of cell populations (‘spillover’), which can be mitigated by focussing on low-granularity cell populations or, if working with a custom signature matrix, adjusting the training phase of the signature generation process (see for example Tosolini et al., 2017, Oncoimmunology).
Comparisons of interest
Depending on the analysis of interest, cell population scores can be compared between samples (to identify differences in cell composition across conditions, tissues, or experimental groups) or within samples (to analyse variations in cell populations within a sample). All tools discussed here allow inter-sample comparisons, but few allow intra-sample comparisons (see Sturm et al., 2019, Bioinformatics for a comprehensive review on inter- and intra-sample analyses).
For example, MCP-counter generates geometric mean scores which represent the relative abundance of a cell type within a sample. These scores are expressed in arbitrary units and do not correspond to actual cell population proportions; therefore, MCP-counter can only compare scores between samples.
Conversely, CIBERSORT (for use in academic research only due to licensing restrictions) or quanTIseq are true deconvolution methods which model gene expression in a sample as the weighted sum of the expression profiles of its cell populations. Thus, they provide absolute fractions of each cell type, allowing to quantify the proportion of cells within a sample that belong to a specific population or remain uncharacterised.
Concluding remarks
Cellular deconvolution is a powerful tool to elucidate the cellular composition of bulk RNA-seq samples. However, given the increasing number of tools available, choosing the appropriate method needs careful consideration of the key factors discussed above.
For example, to compare differences in CD4+ T cells between healthy and infected HIV patients, xCell, quanTIseq or CIBERSORT could be used. MCP-counter does not include a signature for CD4+, while the remaining tools listed here are mouse-specific. However, to estimate the CD4+/CD8+ ratio, it is necessary to estimate the abundances of both T cell populations within the same samples. Therefore, the selected tool must support intra-sample comparisons, so only quanTIseq or CIBERSORT would be suitable tools for this application.
If you wish to learn more about cellular deconvolution, we have an example cellular deconvolution analysis report which you can access by completing the form below:
About the author
Dr Hannah-Louise Hayman is a Bioinformatician and holds a PhD in Precision Medicine from the University of Glasgow, where she studied interactions between the immune system and colorectal cancer. Hannah-Louise joined Fios Genomics in 2023 and has since successfully led many projects for our clients. To discuss how the team at Fios Genomics could help with a cellular deconvolution project, contact us today!